The Social Impact of Data Science
Putting Data Science in context with its growth, its potential, and why it's important to win the race to bend it towards positive impact.
Gabriel Editorial Note Here — Hiya! Thanks for signing up! I'm still playing around with the content, format, length, style, etc. I'd love to have any feedback on these or any other topics; feel free to let me know! What's more — if you like this newsletter, please help it grow by passing along to friends and sharing it on social media. Every shared link helps!
It Started with Text Games
When I was a kid, I remember booting up the family computer in the office to play "Vampire's Castle," a 1984 text-based game where you had to solve the challenges by typing in commands to the interface (you can still play it here). Much of the experience was trial-and-error, as you tried different words "LOOK," "INSPECT," "GO WEST" and would be lucky if 1 in 10 did anything. It was frustrating, and I still remember getting caught up not knowing what a parapet was. The game's vocabulary was pretty limited — and it turns out it was only about words that it "knew" as commands. I remember thinking at the time that it would be so much better if it could just understand what I wrote in actual sentences.
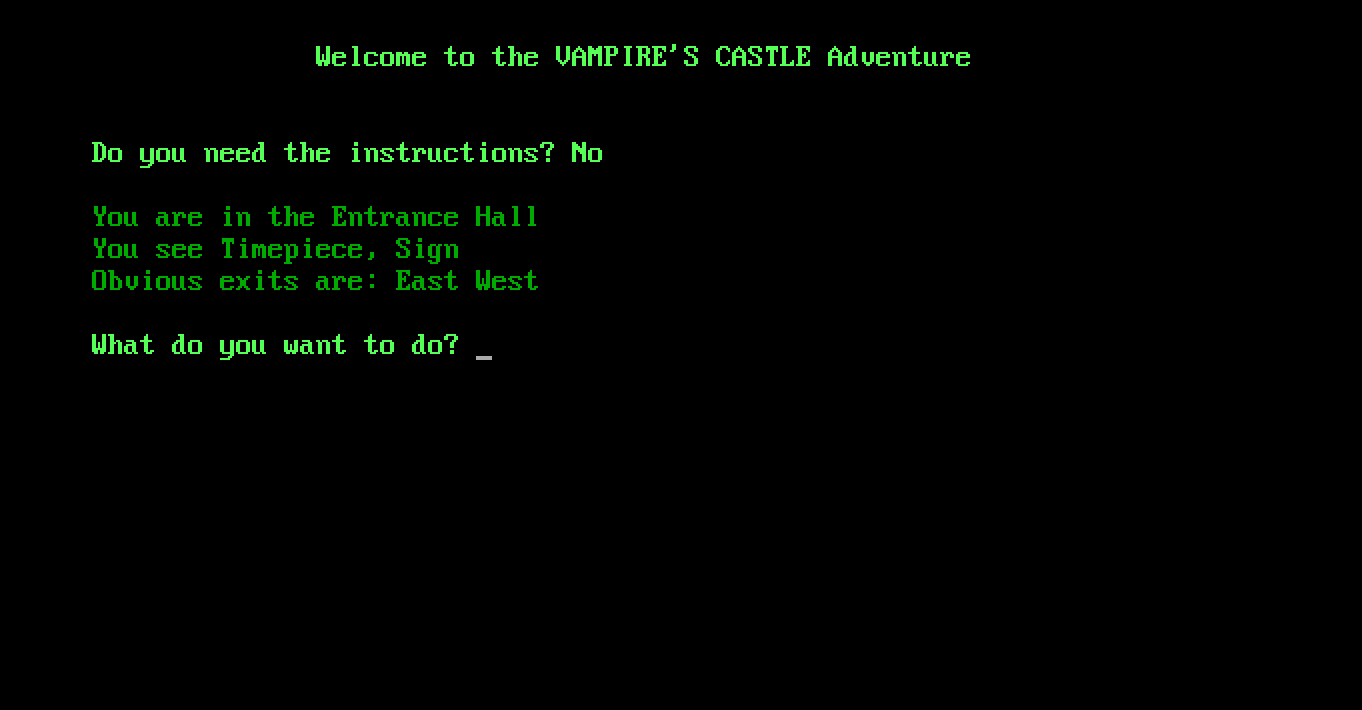
It turns out, I just had to wait a few years for data science to mature. Computing has come a long way in a short amount of time these last few decades.
Gatekeeping Data Science
I find the "science" part of "data science" can be a bit of an unfortunate gatekeeping term. It keeps people out by making it sound like it's only done in cleanrooms or academic chalkboards.
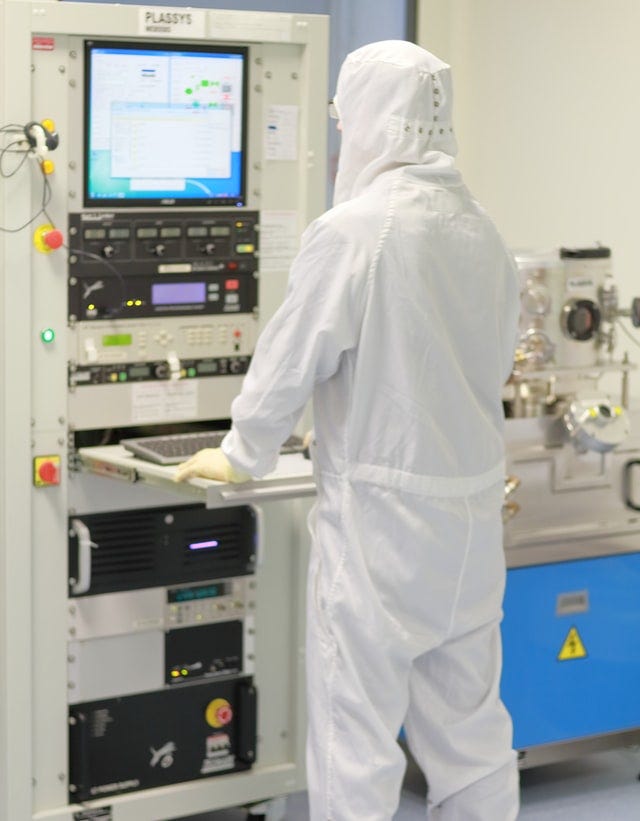
But data science has become the de facto way to look at the intersections of people, information, data, and technology.
And a lot of people with vastly different skills are needed to work in this space.
Data science already has a considerable effect on people's lives (see: advertising, supply chains) and will have an even more significant impact moving forward (see: healthcare, climate change).
It's going to be part of the conversation. But that doesn't necessarily mean it's always going to be a welcome part of the conversation. Examples in just the last week of influence-gone-bad: Facebook's Face Recognition and Zillow's stock crash of 30% (where the CEO put much of the blame on the data science team).
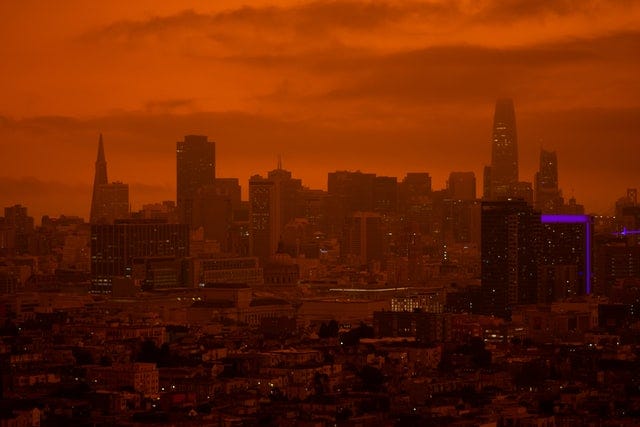
The Social Impact Part
It's our opportunity (and responsibility, some would say) to use these advances in a way that provides overall benefits to society. And it's a race to the finish line.
It's a race to see how quickly we can adopt these technological advances into something beneficial before time runs out. And by time running out — it's not just a global apocalypse spawned from advancement (though, that's there too), but a race against time to provide help to those who have the most to benefit from it. For example, can we develop life-saving vaccines (with computing power assistance) to prevent hundreds of thousands of deaths? Can we use computers to help us design climate modeling to help us survive the next century? Can we use algorithms to help us design economic systems to pull people out of extreme poverty?
In other words, can we use computers to save lives and improve the human condition?
Or, at the very least, can we use computers and algorithms and data science to positive effect before we use it only to build an authoritarian global surveillance state and possibly destroy society in the process?
Unfortunately, you might say that these two ends are somewhat unrelated or at least possible at the same time. This take is probably correct — we are going to see a lot of negative social impact examples with a few outstanding good ones.
But I'm also not convinced that they are entirely mutually exclusive. The power of scale of data science, machine learning, and AI seems to imply that the next decade will be critical in pointing this science in the right direction before it becomes too unwieldy to backtrack.
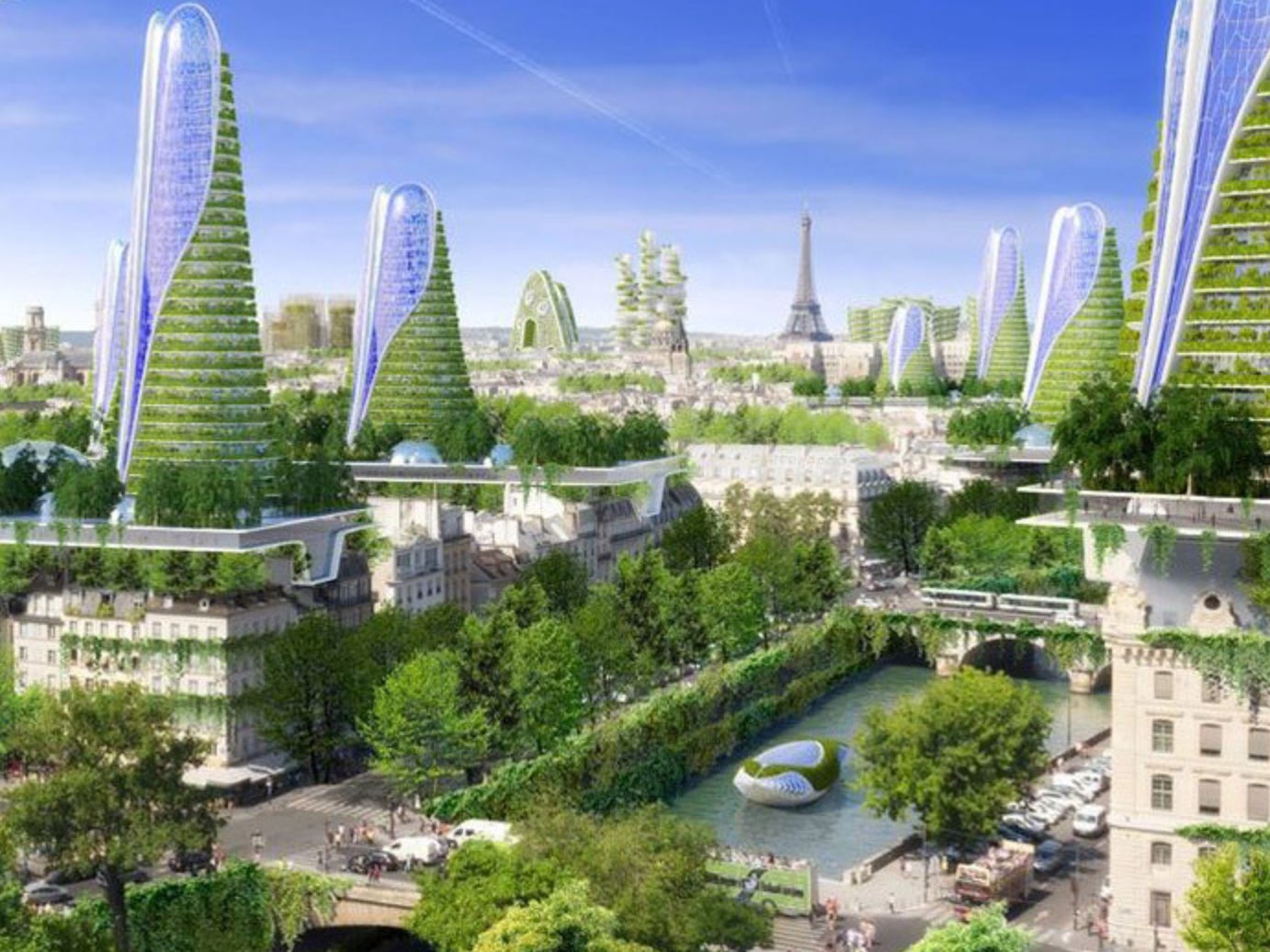
A Theme of Social Impact and Data Science
In the coming months, one of the themes I intend to cover will be around data science and social impact in more specifics — both good and not-so-good examples, how we ought to think about evaluating them, etc. Let me know if there's a particular area of interest for you!
What Else I'm Reading
Exclusive Interview with Billionaire Charlie Munger on Controversial UCSB Dorm | 2021-11-01 | Architectural Record — I'm learning everything I can about this weird dorm story. It's got a lot of stuff to hold your interest: a 97-year old amateur billionaire designing a 4,500-room dorm, and only 6% of the rooms will have windows. It seems like I wasn't the only one watching Squid Game.
What I learned from a year on Substack » Nieman Journalism Lab — I mean, obviously I'm reading this, having just started my own Substack newsletter!
Flow — Saw the recent passing of Mihaly Csikszentmihalyi. My dad gave all of us kids a copy of 'Flow' when I was in high school, and we talked about the concept all the time. It's stayed with me, thinking through careers, hobbies, and more.
The 37-Year-Olds Are Afraid of the 23-Year-Olds Who Work for Them - The New York Times — I think every friend group I have sent this article around, and I'm still deciding what I think of it.
What happened when a ransomware attack hit Pottawatomie County, Kansas. — Close to home for me.
Person Furious That Someone Right Click Saved Their Precious NFT — I'm learning a little more about NFTs for some reason, and this story confirmed a lot of my thinking on the topic to date.
Inside the jaw-clenching world of cricket fighting in China: NPR — Oh. Who knew!
Cheers,
Gabriel