Three Tips for Organizing Data Science Teams for Impact
How to think about culture, timelines, and scoping.
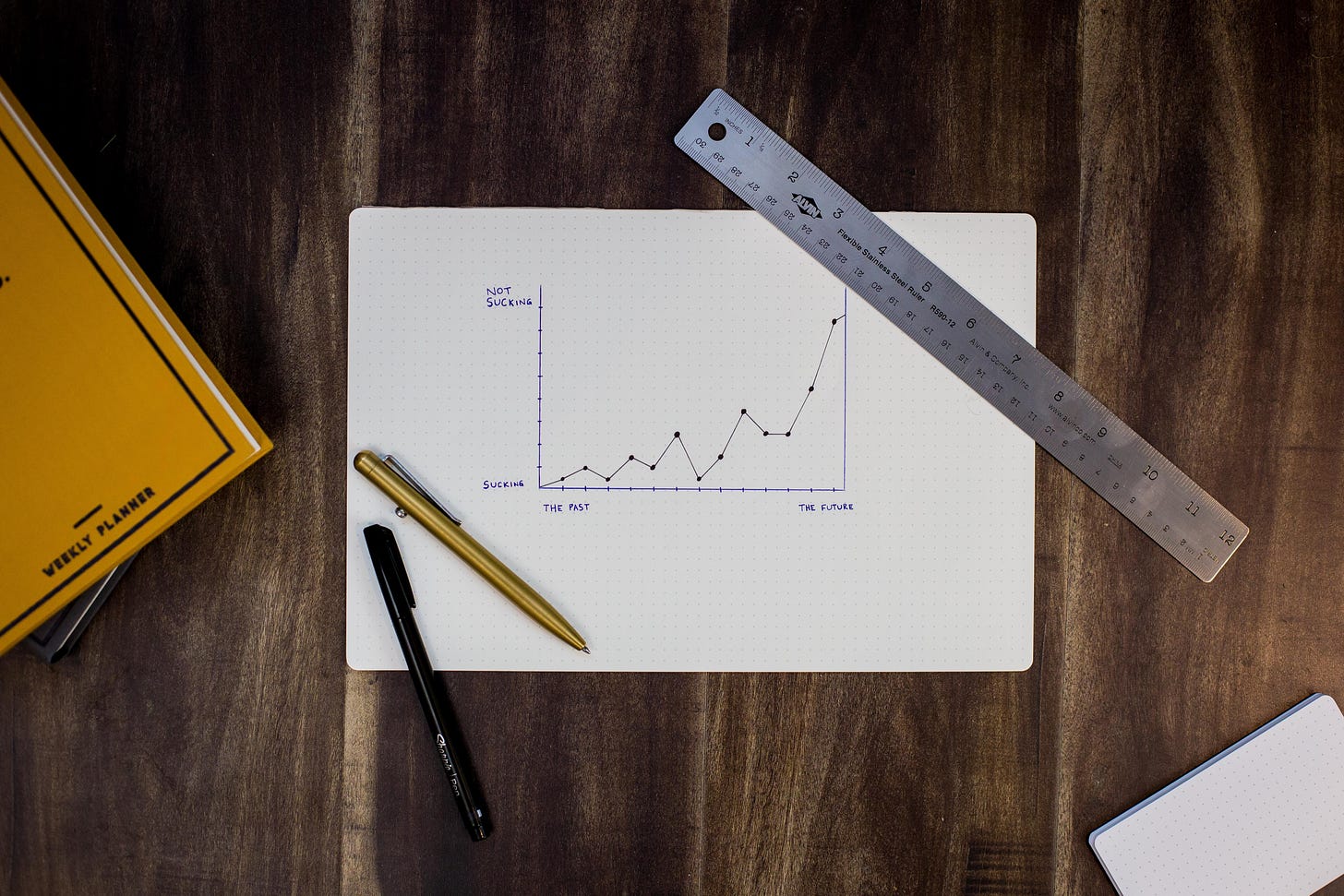
I've changed industries a couple of times and have had the good fortune to be a part of several teams looking to build their data science and AI capabilities.
I've seen a few challenges in different organizations as they start at varying phases of their data science journey, so this edition might be a little more geared towards the data nerds and product folks on the list than most people. Still, I think it's also generalizable enough for most folks thinking about technology intersections.
Why Data Science
Before getting started down the road of data science, modernizing technology stacks, and integrating the latest cloud architectures with your team's data — it's essential to take that giant step backward and ask — WHY?
In other words — how will data science improve your product, your team, your impact? How will it change your approach, and how do you as a team manage your processes? What are you hoping to get out of it? How are you approaching your investments in terms of time, personnel, resources, and more?
OK, once you've started giving yourself a heart attack with those easy ones — I usually sketch out three broad areas of thinking.
Culture — Align within your organization
You shouldn't be chasing data science because it's new and shiny — even if it is. Too many organizations start from the fantasy of solution orientation and are quickly disappointed when it's challenging to match that enthusiasm from the needs-assessment side. Your teams don't want data science if they are already tapped out on their bandwidth and energy, wouldn't see the benefits to their tasks or project output, and aren't involved in the journey.
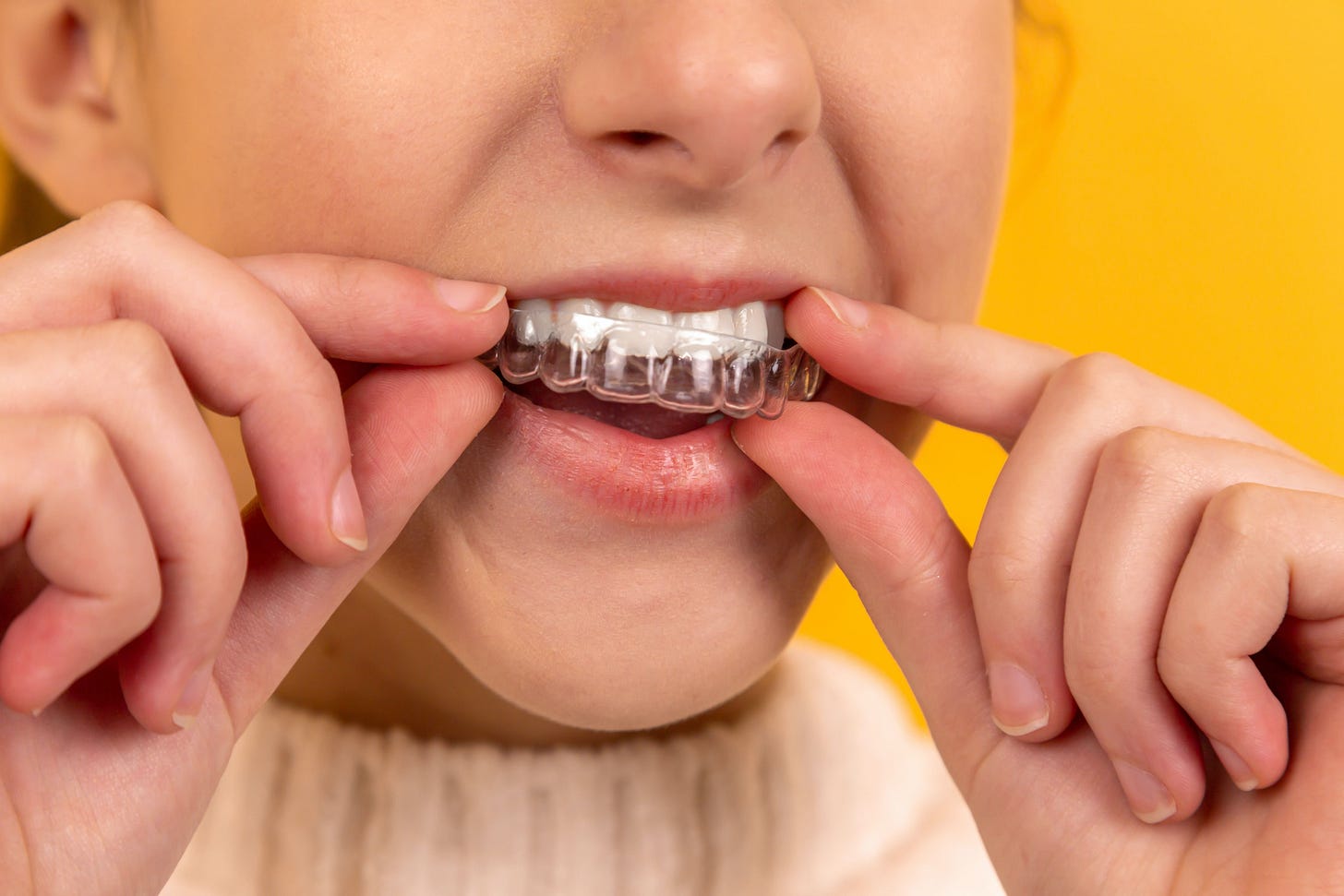
You have to line up the context of where your organization is at — the expertise, the experience, the priorities, and the personnel, and have them all facing in more or less the same direction to take on data science.
This can be done through sponsored trainings, fellowships, internal cohorts, even internal hackathons, and half-day workshops are good ways to get the right people to the table and try some of these things on for size. This way, you're not only building skills, but you're also building the networks, and through that, you're building the internal culture that tethers your teams to new ways of thinking and problem-solving around data science.
One way to do this is through data science competitions like those run by Kaggle (and there are more than just Kaggle).
Timelines — Use time scales, don't fight them
One of the biggest challenges is the disconnect between the overwhelming pace of change we see in this space and the longer time horizons of organizations.
Everyone always feels like they are starting from behind.
But you're not!
"Start where you are, use what you have, do what you can." — Arthur Ashe
Not everything has to be a neural network built with a supercomputer.
Going from a paper-based survey collection process to an Excel spreadsheet can be an absolute game-changer. We can build on existing solutions and translate that practice where and when appropriate.
On the flip side, we also don't want to spend all of our time trying to formulate the one-big-platform-to-rule-them-all. When we do this, we start to enter Everything Pig territory, and that's a no-no.
Instead, we want to think about building in small, incremental, but ultimately valuable phases. This drawing from Henrik Kniberg that I came across a few years ago is perfect. I think the reality of the top-down approaches that spend years on a path without building functional iterations along the way are much less cheery than the top row in this drawing — they often get canceled before it even comes to this.
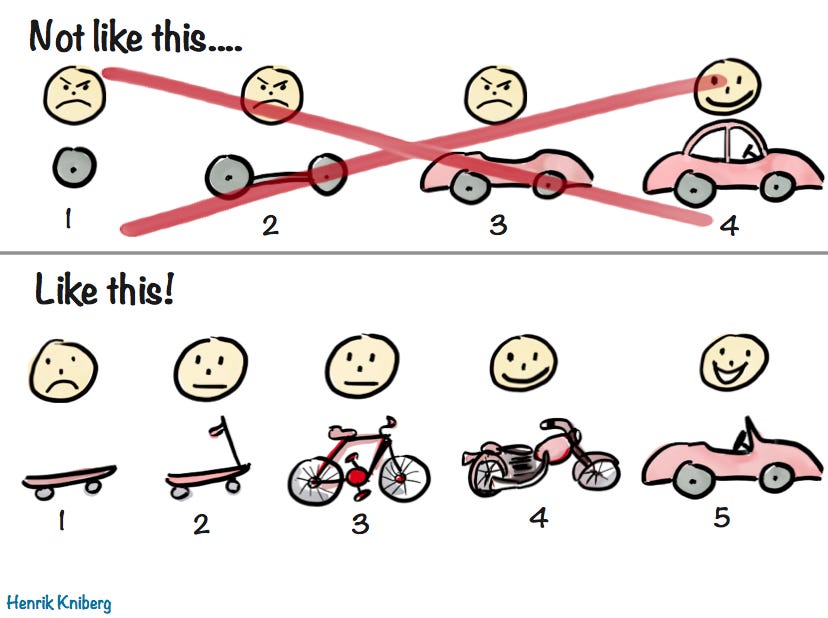
My favorite recent example was an app project that I led where we built a smartphone app to detect mosquito eggs. It started out simply — just in recognizing small black dots from a photo. Eventually, it grew to encompass different types of images, user controls for various conditions, multi-language support, training, and more. But we didn't do it all at once.
Scoping — Technology is hidden, and should be
When I hear a team start by saying, "We really need to data science this..." — I know that we've already started too late.
Technology is often just a means to an end.
When you read the reports of organizations and governments, you find that you don't need to invoke technology to talk at all about impact. What I mean is that when you're reading about healthcare initiatives, access to clean water, improved education, etc., what you see is the focus is on improved access to resources, greater financial freedom, or higher test scores. You don't necessarily hear about health records systems, supply chain databases, or learning management software.
When I hear someone say the scope of work doesn't have anything about technology — my first thought is usually one of relief, as it means the project isn't hobbled by being forced to go down a particular technological path. This way, we are free to build the product or service to most effectively meet the needs of the team.
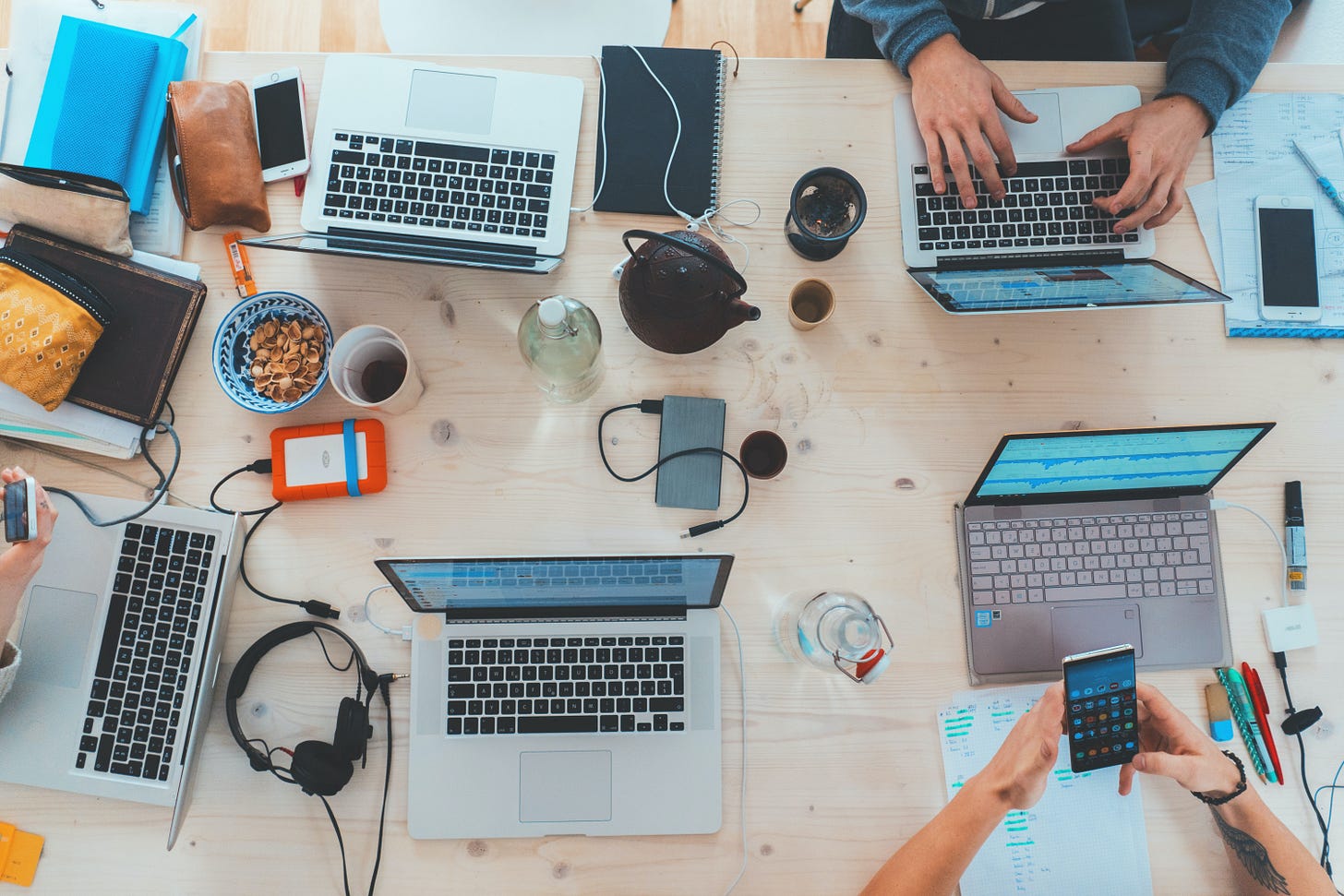
Thoughts
By starting small, with the right team, and building upon small but essential wins, you can begin to see some very encouraging progress.
And so, even though it won't be easy, I'm optimistic about our opportunity to be that force for good, design and develop cooperative systems, and be part of an organization that helps make a positive impact outside the scope of technology — using data science and beyond!
What Else I’m Reading
The Great Switch: distributed work first – James Governor's Monkchips — Good lessons about thinking through distributed work.
The Smart Nonprofit: Staying Human-Centered in An Automated World by | Staying Human-Centered in an Automated World — Lots of similar thinking from this article (and excerpted book).
Shopify’s Evolution – Stratechery by Ben Thompson — I love Ben Thompson’s thinking on most topics, and really provides the best ways of thinking about this sector.
Elon Musk Says SpaceX Starlink Satellite Internet Is Active in Ukraine — Comparing to my previous example.
Bionic eyes: Obsolete tech leaves patients in the dark - BBC News — Well this is nice and dystopian.
Why Africa will be the world's fastest growing video game market — Quartz Africa — Bet you didn’t necessarily see this one coming.
Thanks for reading,
Gabriel
—
GoodTinker is a weekly email from Gabriel Krieshok about technology, design, and social impact. If you’ve enjoyed this edition, please consider forwarding it to a friend. If you’re reading it for the first time, consider subscribing (it’s free!).